Academic Journal of Applied Mathematical Sciences
Online ISSN: 2415-2188
Print ISSN: 2415-5225
Print ISSN: 2415-5225
Quarterly Published (4 Issues Per Year)
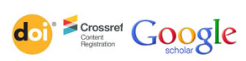
Archives
Volume 6 Number 2 February 2020
M2D-QPCA: An Improved Quaternion Principal Component Analysis Method for Color Face Recognition
Authors: Lili Song ; Kaisong Sun ; Minghui Wang
Pages: 5-14
DOI: doi.org/10.32861/ajams.62.5.14
Abstract
Principal component analysis (PCA) is one of the successful dimensionality reduction approaches for color face recognition. For various PCA methods, the experiments show that the contribution of eigenvectors is different and different weights of eigenvectors can cause different effects. Based on this, a modified and simplified color two-dimensional quaternion principal component analysis (M2D-QPCA) method is proposed along the framework of the color two-dimensional quaternion principal component analysis (2D-QPCA) method and the improved two-dimensional quaternion principal component analysis (2D-GQPCA) method. The shortcomings of 2D-QPCA are corrected and the CPU time of 2D-GQPCA is reduced. The experiments on two real face data sets show that the accuracy of M2D-QPCA is better than that of 2D-QPCA and other PCA-like methods and the CPU time of M2D-QPCA is less than that of 2D-GQPCA.