Academic Journal of Applied Mathematical Sciences
Online ISSN: 2415-2188
Print ISSN: 2415-5225
Print ISSN: 2415-5225
Quarterly Published (4 Issues Per Year)
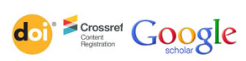
Archives
Volume 7 Number 4 October 2021
Building a Predictive Model for Gynecologic Cancer Using Levels of Data Analytics
Authors: Faisal Alamri ; Ezz H. Abdelfattah ; Khalid Sait ; Nisreen M. Anfinan ; Hesham Sait
Pages: 192-197
DOI: doi.org/10.32861/ajams.74.192.197
Abstract
The four levels of data analytics techniques (descriptive, diagnostic, predictive, and perspective) were used as a methodology. We also used data mining techniques to predict Gynecologic cancer before any lab test or surgical intervention. Influencing and associating between factors are used to cover hidden relationships or unknown patterns. We focused on three types of Gynecologic cancer (cervical, endometrial, and ovarian cancer). We collected an initial examination of 513 (228 benign and 285 malignant) patients from King Abdulaziz University Hospital (Saudi Arabia). Data were collected during the period of 16 years (2000-2016). After examining many models, we found that the classification trees C5 and CHAID beside the Support Vector Machine (SVM) algorithm give the highest accuracy, with the values of 87.33 %, 79.53%, and 78.36 % respectively. The sensitivity and specificity were found to be 86.18 % and 89.00 % for C5. The corresponding values for CHAID were found to be to equals to 82.20 % and 76.72 % while for support vector machine (SVM) the values were found to be 83.74 % and 77.10 %.
The Modified Increment Method for Eigenspace Model
Authors: Chunjie Wei ; Jian Wang
Pages: 187-191
DOI: doi.org/10.32861/ajams.74.187.191
Abstract
Eigenspace is a convenient way to represent sets of observations with widespread applications, so it is necessary to accurately calculate the eigenspace of data. With the advent of the era of big data, the increasing and updating of data bring great challenges to the solution of eigenspace. Hall, et al. [1], proposed that the incremental method could update the eigenspace of data online, which reduces computational costs and storage space. In this paper, the updating coefficient of the sample covariance matrix in an incremental method is modified. Numerical analysis shows that the modified updating form has better performance.